WELCOME
Over the last few years, significant improvements in the fields of artificial intelligence (AI) and machine learning (ML) have had a profound impact on numerous scientific disciplines. In this context, the development of AI and ML-based scientific applications has ushered in an era defined by an unprecedented ability to analyze massive scientific datasets and uncover patterns that may elude human observers, revolutionizing the way we approach and tackle complex challenges across diverse domains, from Mathematics, and Statistics to Physics and Biology.
In many sub-fields within these scientific disciplines, AI and ML methodologies constitute nowadays a common methodological resource around which interdisciplinary collaboration can flourish. This convergence of expertise and technology allows scientists to foster rich ecosystems of interdisciplinary cooperation, combining insights and approaches from different domains to tackle complex problems with unprecedented efficacy.
The XIth Edition of the BIFI International Conference 2024, hosted at the Institute for Bio-computation and Physics of Complex Systems, at the University of Zaragoza, will gather under the same umbrella a wide variety of leading scientists working in an array of fields in Physics, Biology and Statistics where the development and applications of AI and ML-based Methodologies are pivotal. All topics broadly related to AI and ML applications in these disciplines are welcome to the meeting, where scientific contributions will be organized according to the following tracks:
AI: Methods and Applications
The transformative potential of artificial intelligence (AI) resides in its remarkable capacity to delve deeply into complex datasets of diverse structures, encompassing continuous multidimensional data as well as unstructured content like text, images, or videos. The development of new models and enhanced algorithms to improve the diagnostic and predictive capabilities of AI-based pipelines constitutes a fundamental priority in the field.
In this track we welcome contributions spanning both Methodological developments in AI and ML, as well as their application in broad data-intensive domains. Topics of interest include Unsupervised Learning, Deep Learning, Natural Language Processing, and Computer Vision, among others.
AI and Statistical Modelling
In many cases, the lack of transparency and interpretability of ML techniques and models are mentioned as a cardinal feature of these transformative techniques that limits their applicability potential. For these reasons, the combination of AI and ML methods with statistical modeling is emerging as a powerful methodological resource, aiming at combining the interpretability of statistical methods with the diagnosis and prediction capabilities of AI and ML models.
In this track, we welcome contributions to the interface between Statistical modeling and AI, including, but not limited to Stochastic Models, Bayesian Inference, and Supervised learning Methods, among others.
AI Applications in Physics
Physics, with its profound insights into the fundamental laws of the universe, has long been at the forefront of scientific discovery. However, many phenomena within the physical world, from weather patterns to subatomic interactions, exhibit complex, non-linear behaviors that have proven challenging to unravel using traditional methods alone.
Recently, AI and ML-based computational Methods have emerged as a powerful ally in our quest to comprehend these enigmatic systems. This session invites contributions that explore the synergy between AI and physics in deciphering complex systems.
We welcome research that dives into a wide range of topics, including but not limited to complex systems, material science, fluid mechanics, quantum physics, environmental physics, and interdisciplinary applications, among others.
AI Applications in Biomedicine
Probably one of the most defining features of contemporary biomedical research is its expanding ability to produce quantitative data with ever-increasing quality, resolution, throughput, and volume. From the revolution of next-generation sequencing techniques to the emergence of data-intensive technologies in structural biology such as cryo-electron microscopy, the significance of dry-lab tasks in biomedical scientific workflows is steadily rising, in line with the growing dimensionality, volume, and complexity of the data we generate.
In this context, the application of AI and ML Methods has proven to be indispensable, catalyzing a number of transformative advances. This session invites contributions that explore the synergy between AI and Biology in deciphering Molecular Biology and Systems Biology alike.
In this track, we welcome research on a wide range of data-intensive biological fields, including but not limited to omics-data analysis, advanced microscopies, and protein structure, among others.
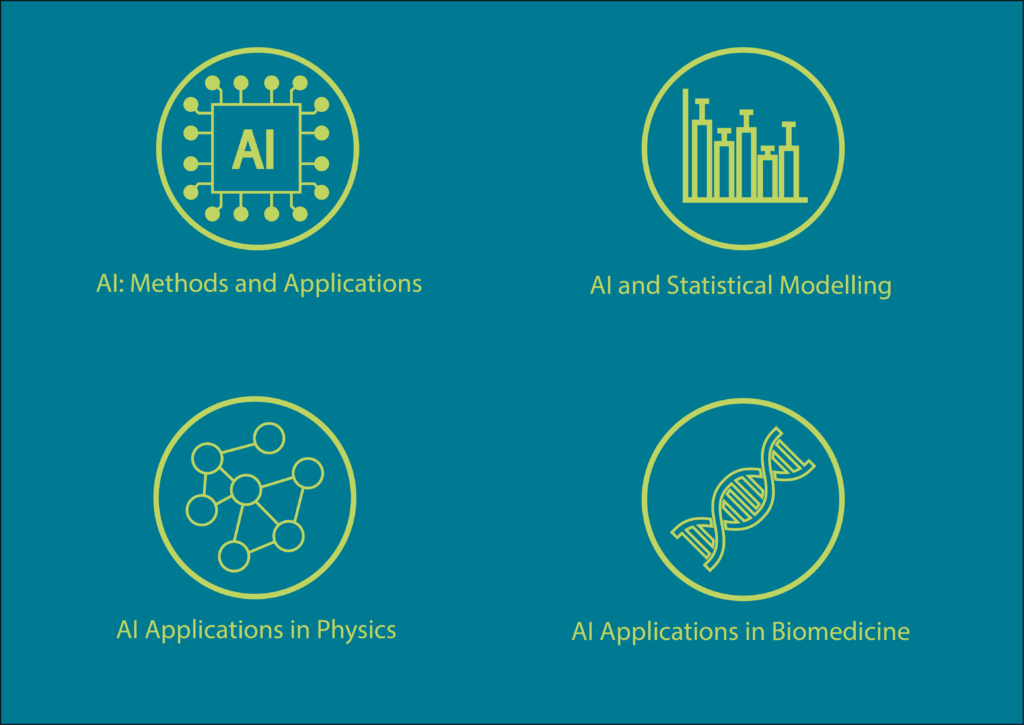